Fast Forward Labs' Jessica Graves on Farming Big Data
The research analyst explains why we can't afford to ignore numbers.
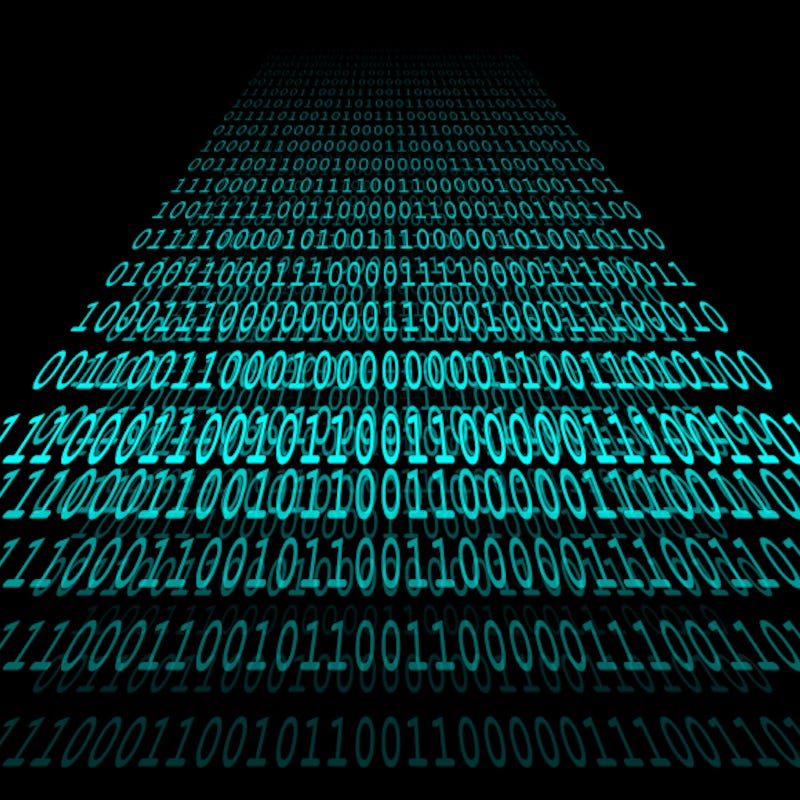
The race to collect data is so 2010. The next race is to understand it. And Fast Forward Labs, a research firm founded by data scientist Hilary Mason (formerly of Bitly) is out in front. The company works with all kinds of businesses, big and small, and tries to anticipate how emerging technology trends will impact their respective industries. They are number crunchers with unbelievably powerful crunching machines. Analyst Jessica Graves operates these machines, using “the least understood computational capabilities” to discover and understand nuances.
To say that she is detail oriented undersells it. She can not only find a needle in a haystack, but sort the hay. Inverse spoke with her about using big data and learning how to communicate what it tells us without missing the point.
What sets Fast Forward Labs apart from other research firms, which may be bigger and able to allocate more resources to projects?
Our reports and prototypes are built in heavy collaboration with our in-house design team. We are very careful about how to visually represent abstract concepts to translate the point further. Our overviews bridge non-technical and technical team members, but also give the tech team a useful working guide to implementation.
Fast Forward Labs also gives special weight to the ethical implications of deploying certain types of technology, which I’ve never seen in industry before. Most people won’t want to point out to their clients what could go wrong with widespread use of a new technological capability.
As I understand, the company has a strong interest in analyzing and using big data in its projects. For our readers who might be unfamiliar, can you describe what “big data” means, and how you and your company use and interact with this type of information?
Many companies have data streaming in as a side-effect of their business. What makes it “big” data is that it’s too difficult to look at directly and make sense of without taking serious care of which algorithmic and statistical paradigms come into play. This kind of technology changes quickly, and the combination of prototyping, reporting, and consulting means that Fast Forward Labs can keep clients up to date at any given time.
Why has big data become such an important part of how businesses operate these days? Was there a particular moment when the potential for harnessing data took a leap, or was it a gradual progression?
Storage capabilities leaped over time. If you think in terms of the web, there’s a negligible hardware cost difference per user between storing data about 10 thousand users or 10 million users. I just read a piece about the development of tracking cookies in the ‘90s which made it easier to get a better sense of unique visitor information on websites. It’s cheaper than ever to store information about what consumers are doing on all parts of the web, down to how many seconds a user spent hovering over a candid celebrity photo.
Who needs big data? What sectors or industries rely on it the most? What are some particularly innovative or surprising ways data can be used by people and businesses?
It’s difficult to specify an industry. Any business that makes decisions that can be validated in a quantitative way can benefit from data. This type of research allows us to parse through previously incomprehensible data stores to bring about innovative health outcomes… or find the cutest cat on the Internet. There are many reasons to use and not use big data, and we do pay close attention to ethical consequences of algorithmic developments.
Are there any emerging trends in data science that you and others at Fast Forward Labs have noticed? What are they?
Deep learning is a big one. For example in fashion, one of the holy grails of ecommerce is to allow customers to search for items by uploading an amateur photo of something they’re interested in. It’s hard to automate this kind of image processing without neural networks, and there have been interesting papers and startups springing up around this.
Not to ask the obvious question, but what’s next?
As a group, Fast Forward Labs is quite interested in statistical natural language processing, particularly finding the most interesting text in a large data set. It’s very exciting on our end to see a renewed interest in artificial intelligence, and emerging products from this field have an unprecedented opportunity for innovation.