Pigeons and Computers Have One Surprising Thing in Common, Study Reveals
“Bird-brained” may as well be a compliment.
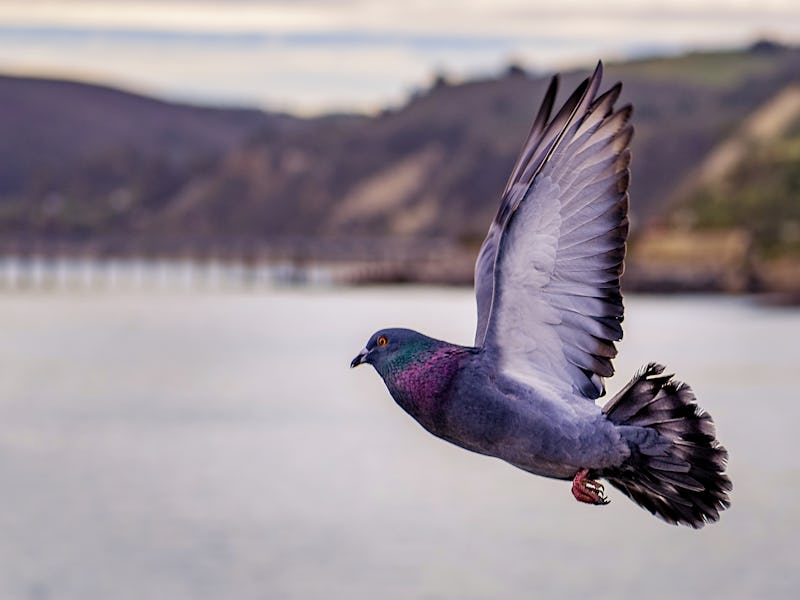
Time and time again, artificial intelligence is heralded for its impressive feats. Computers have beaten humans in dozens of games, crafted essays instantaneously, and even detected cancer with stunning accuracy.
In many ways, AI might seem to surpass the intelligence of humans. But what about pigeons?
The birds’ primary learning strategy is nearly identical to machine learning algorithms on a basic level, according to a new study published in the journal Current Biology.
Obviously, pigeon brains have limitations that computers don’t. But their method of solving challenging problems isn’t all that different from the technique used by some AI systems to pull off superhuman accomplishments.
Here’s the background
Pigeons are actually pretty clever. These bird-brains creatures have expansive memories and a knack for recognizing visual patterns, which is partially why scientists have studied their smarts for decades.
Ed Wasserman, a professor of experimental psychology at the University of Iowa and lead author of the new Current Biology report, has been probing the intelligence of pigeons and other animals for over five decades.
A pigeon.
Wasserman’s work largely focuses on untangling the differences in intelligence between animals and humans. “We've, as a field, always been interested in what makes people special — if, indeed, there is anything that makes us special,” he tells Inverse.
Over the years, researchers have put animals up to increasingly difficult mental tasks. Some studies in Wasserman’s lab have pitted human test subjects against pigeons to see if either excelled at solving certain puzzles. It turns out that pigeons can easily tackle the same challenges that humans can — chipping away at the idea that we’re far more intelligent than other species.
But there’s an important caveat. “If you give animals tasks, and they increasingly resemble the tasks that people solve, the implicit assumption is that they're doing it the same way,” Wasserman says. Instead, that might not be the case.
Digging into the details
While working on a study published last year in the journal Cognition, Wasserman noticed distinct differences in how pigeons approached a problem compared to human subjects.
He challenged both birds and people to gaze at visual patterns and guess how the researchers categorized them. Each pattern featured black stripes of varying widths and angles against a white background. The task seems simple enough, but the challenge comes from how the patterns were grouped.
Instead of separating them by simple logical rules, the researchers sliced them into more arbitrary categories.
An example of pattern categories used in the Current Biology study. The groupings didn’t follow simple logical rules.
“That poses a special problem for both pigeons and people: You can't just look at the orientation of the lines, you can't just look at the width of the lines — somehow you have to combine that information,” Wasserman explains.
Naturally, human participants wanted to decipher the mysterious rules that confined the patterns to their respective groups. But the birds didn’t seem to try that strategy at all.
“Pigeons never showed any tendency to carve the stimuli in terms of the orientation alone or in terms of the width of the lines alone,” Wasserman says. “[But] people did.”
That work raised new questions about pigeons’ learning strategies — and the recent study offers some answers.
What’s new
This time, Wasserman and his team recruited four pigeons to solve a puzzle devised to be even more difficult than the previous Cognition challenge. In fact, this was a bird-only experiment, since Wasserman says it would have been “terribly frustrating” for human participants.
The birds studied similar visual patterns and pressed a button to guess which group each pattern belonged to. If they guessed correctly, they got a treat. And if they pressed the wrong button, the pigeons had to try again until they got the correct answer.
Sure enough, the animals brute-forced their way to victory: they tried and tried again until they got the answers correct.
So how did they do it? Wasserman thinks the birds relied on a strategy called associative learning.
In other words, the birds learned to link each pattern with a positive or negative outcome (getting the answer right or wrong). As time went on, they could better guess if new patterns would belong to group A or group B.
Humans learn this way, too, especially in childhood — because we don’t yet grasp logical rules, we learn to associate stimuli with certain outcomes. Think of how parents cheer a baby on when they say a word correctly or scold them when they throw their toys. This helps children to learn how certain behaviors are encouraged or discouraged.
But as we get older, it’s more common that we rely on declarative, or rule-based learning. Compared to pigeons, our brains are much more inclined to rely on logic and rules we’ve learned conceptually, rather than through trial and error.
In fact, many machine learning algorithms also take the route favored by children and pigeons. When faced with a problem, algorithms test every answer until they get it right — no matter how long it takes.
The big idea
A match between a supercomputer and a pigeon probably wouldn’t be fair.
So does this discovery mean that pigeons could go head-to-head with computers in a battle of wits? Not really.
“When we talk about the pigeon matching wits with AI,we don't mean that they're as smart as AI — in all likelihood, [birds] don't have the capacity,” Wasserman notes. “But the mechanism behind it seems to be the same.”
This finding checks out, Christopher Kanan, a professor of computer science at the University of Rochester who did not work on the new study, tells Inverse.
Associative learning is a pretty common technique in machine learning, one of Kanan’s areas of expertise.
It’s often used in a subcategory of AI called supervised machine learning, which “is trained in a way where we tell the systems: Here's an input or a stimulus … and then here's a desired output,” Kanan explains. “And if the machine does not predict the correct output, we kinda penalize it to make it try to get the output correct the next time.”
Just like the pigeons, machines try over and over again to get the answer right. That’s why associative learning can be so powerful for algorithms sifting through unstructured data, Kanan says.
Trying every solution individually isn’t an efficient process, but high computing power can speed up the time it takes to learn how to complete a task.
“You may need vastly more computational resources, vastly more experiences to achieve that level of performance [of] a human,” Kanan says.
He cites the example of the OpenAI Five program designed to defeat players in the online game Dota 2: the computer required 45,000 simulated years of experience in order to beat the top human players in the world.
So even if pigeons are capable of thinking like algorithms, they probably won’t be challenging computers anytime soon — unless they develop their own superhuman ability to sort through copious amounts of data.
This article was originally published on