Astronomers Use a New Method to Identify Eight Candidate Alien Signals
A machine learning algorithm just spotted eight interesting signals in SETI data, and it weeded out millions of false positives.
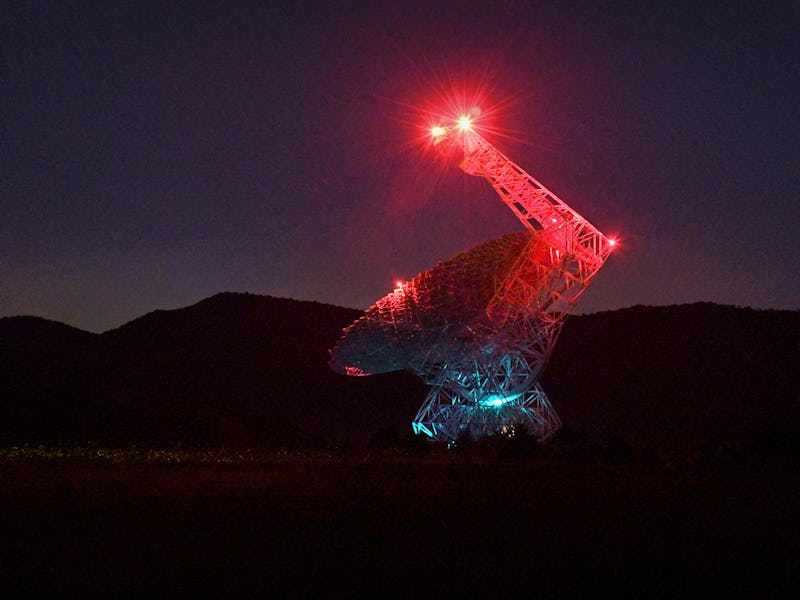
Imagine the moment in Contact when Jodie Foster hears an alien radio signal coming from the Vega star system. Now imagine that Jodie Foster is an AI.
That might be one version of the future for the search for extraterrestrial intelligence (SETI), a decades-long effort to find cultures on other planets by looking for what SETI researchers call technosignatures: everything from alien radio messages to giant star-enclosing megastructures. University of Toronto machine learning researcher Peter Ma and his colleagues recently trained a machine learning algorithm to comb through radio astronomy data, searching for potential alien signals — and it spotted eight interesting signals that previous searches missed.
Ma and his colleagues published their study in the journal Nature Astronomy.
What’s New — Ma and his colleagues turned their machine-learning algorithm loose on about 480 hours of radio astronomy data from the Green Bank Telescope in West Virginia, which had been gathered as part of the Breakthrough Listen initiative, a privately-funded search for alien communications. They had trained their algorithm on a mixture of real-world radio signals and simulated extraterrestrial chatter, to look for what SETI researchers call “signals of interest.”
A signal of interest is anything that’s not obviously a human radio signal (radio astronomers have to weed out a lot of interference from human gadgets) or bursts of radio waves from dead stars or other natural phenomena. The alternative is that it might be a signal from an alien civilization or some kind of natural phenomenon that we haven’t discovered yet.
In the end, Ma and his colleagues’ algorithm flagged eight “signals of interest” that previous studies hadn’t spotted in the data. But it took some work to get there.
The Green Bank Telescope (R), the largest of eight radiation detecting dishes at the Green Bank Observatory in Green Bank, West Virginia, points into space on October 7, 2021. - The Green Bank Telescope s the largest fully steerable radio telescope in the world. Eight dishes of different sizes at the observatory detect radiation from objects in space in the form of radio waves. Despite its population of under 200 people and remote location among rolling hills, dense forests and farms about four hours' drive from the capital Washington, Green Bank is a place of international fascination. (Photo by ROBERTO SCHMIDT / AFP) (Photo by ROBERTO SCHMIDT/AFP via Getty Images)
On the first pass through the data, the algorithm flagged a whopping 3 million signals of interest, out of about 115 million snippets of data (each snippet represented a few Hertz of radio bandwidth and a short period of time).
“You can imagine the data we’re working with as an extremely long ‘image,’ so to speak. It's a 2-D grid,” Ma tells Inverse. “The data is 16 x 300 million pixels. To work with this data with deep learning models we chop up this super long “image” into chunks of 16 x 4000. We then ask the model, ‘Is there something interesting in this small window of 16 x 4000? This chunk is a snippet.’”
When the algorithm flagged a snippet as interesting, it included a score measuring how confident it was that the event was really worth looking at more closely. Ma and his colleagues tossed out everything with a score below 90 percent, then they weeded out any frequencies that had too many signals — a sign of interference from human radio — and the frequencies that were already known to be clogged with radio interference. That left 20,515 snippets worth a second look.
While 20,515 sounds like a lot, Ma and his colleagues say it’s about 100 times fewer snippets than previous analyses of the same dataset, which used more straightforward computer programs like turboSETI, had flagged. According to Ma and his colleagues, that suggests that their machine learning algorithm is better at weeding out false positives: things that look like possible alien signals but almost certainly aren’t.
A close look at the remaining 20,515 snippets turned up a total of eight that previous studies hadn’t identified — eight enigmatic radio signals that might, or might not, be messages (or maybe just stray vintage sitcoms) from an alien culture.
Digging into the details — The eight “signals of interest” the algorithm flagged came from five nearby stars, between 30 and 90 light years away. All five signals had the telltale signs of something that might be alien radio waves. First, the signals were broadcast on just a particular, narrow band of frequencies. And second, the signals’ wavelength seemed to wobble a bit thanks to something called the Doppler Effect, in which radio waves get stretched or compressed as the sender moves farther from, or closer to, the receiver.
“If a piece of extraterrestrial technology was emitting radiation at a fixed frequency from a platform that is rotating (like a planet) or moving under Keplerian motion (like something orbiting a star), it would be accelerating relative to our receiver and thus should exhibit Doppler drift,” says Ma.
In other words, Doppler drift is a clue that a signal may be coming from a planet and not from the star itself.
Everybody stay calm — This doesn’t mean that Ma and his colleagues, and their machine learning algorithm, have found eight alien radio messages. What they’ve found are eight radio signals that they can’t immediately classify as interference from human radios or astrophysical events.
“Given that the main goal of this work is to apply a machine learning technique to identify signals with a specific pattern, we do not attempt to make a definite conclusion of whether these eight signals are genuinely produced by extraterrestrial intelligence,” write Ma and his colleagues. “We encourage further re-observations of these targets.”
The signals may turn out to be a type of Earth-based radio interference the algorithm and the researchers didn’t recognize, or they may turn out to be a new type of astrophysical phenomenon — which would be very interesting in its own right. That’s happened before; the astronomers who discovered pulsars also briefly considered the possibility that they might have found aliens.
When astronomers pointed Green Bank’s antenna at the five stars in question a second time, they heard nothing. That’s not uncommon in SETI; one of the most famous detections ever, the “Wow” signal, also never repeated. And SETI researchers often identify signals of interest that turn out to be nothing, can’t be identified, or are never heard from again. We may never know for sure.
Here’s the background — Most Breakthrough Listen data gets processed by a computer program called turboSETI; modern astronomers in most fields, SETI and otherwise, rely on computer programs to process the massive amounts of data they have to deal with. These pipelines are programmed to look for certain characteristics in the data, instead of learning and extrapolating from real-world examples.
“TurboSETI is a traditional algorithm, in that there is no component that learns from the data,” says Ma. “My machine learning work differs in that it learned to do all of this from scratch from the data I fed it, with no need to program its instructions a priori.”
Ma and his colleagues trained their AI by showing it lots of examples of human radio interference and astrophysical signals. They also throw in a few simulated alien signals to show the algorithm what an actual detection might look like. Over time, the algorithm learns what kinds of things are likely to be human radio signals, what’s likely to be a distant star, and what might be the real deal. Because it learns from experience instead of just being handed a set of criteria, the machine learning algorithm can, in theory, be more flexible and make connections a simpler program might not.
And Ma and his colleagues say it worked pretty well.
“We find that our model is successful in returning fewer false positives and more convincing signals of interest,” they write.
GREEN BANK, WV-JUNE 07: The Robert C. Byrd Green Bank Telescope as seen at night in Green Bank, West Virginia on June 7, 2019. Note that the array of small green dots in the lower right portion of the photo are of blinking fireflies. The Robert C. Byrd Green Bank Telescope in Green Bank, West Virginia is the world's largest fully steerable radio telescope. The telescope is taller than the Statue of Liberty and the site where the telescope sits is on a campus that can be visited by the public. (Photo by Michael S. Williamson/The Washington Post via Getty Images)
What’s next — Ma and his colleagues hope to try their algorithm again on more Breakthrough Listen data from Green Bank, Parkes Observatory in Australia, and the Very Large Array in New Mexico.
“These new projects require us to extend this work to even more sophisticated algorithms to deal with multiple dishes, rather than the single dish with the Green Bank Telescope used in this work,” says Ma.
Meanwhile, taking another look at the five stars Ma and his colleagues’ signals came from — and figuring out what created those signals — will be up to SETI researchers.
This article was originally published on