The Words You Use in Facebook Statuses Can Predict 21 Medical Conditions
Terms like "head," "pain," "god," and "tears" are all linked to health conditions.
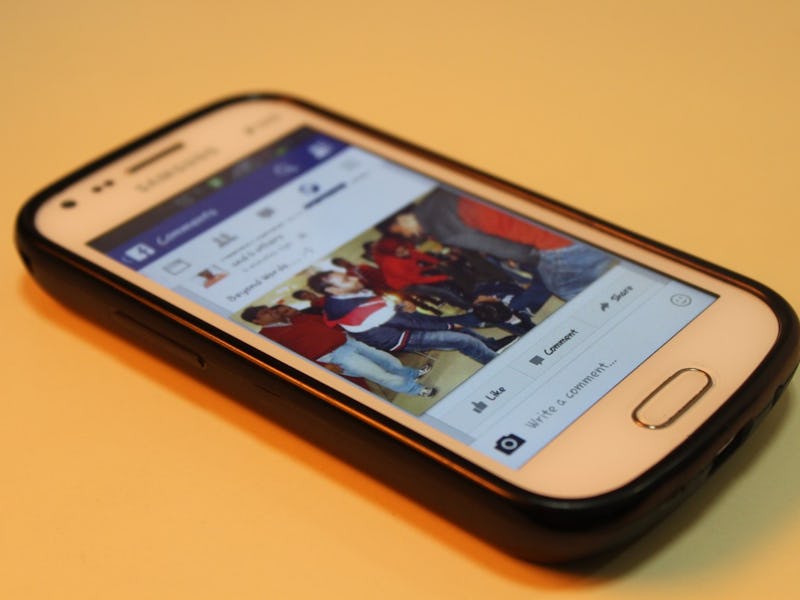
In the eyes of a trained algorithm, Facebook status updates may be more than stray thoughts cast out into an online void. Instead, a team of scientists from the University of Pennsylvania showed that they may represent a “digital health footprint” capable of detecting diagnoses from depression to diabetes with uncanny accuracy.
Using an algorithm described in a paper published Monday in the open-access journal PLOS One, this team reports that language patterns within the Facebook statuses of consenting individuals can be used to predict 21 different medical conditions — including mental health issues and bodily health problems like sexually transmitted diseases. In fact, for 10 conditions, Facebook language proved to be a more powerful predictor than demographic data like age, sex, or race, which have been used to predict conditions and highlight inequities in public health research.
For 10 different diseases, Facebook statuses were better at predicting whether someone had the condition or not.
Facebook statuses were significantly better at predicting cases of diabetes, pregnancy, anxiety, psychoses, chronic pulmonary disease, STDs, drug abuse, collagen vascular diseases, coagulopathy (a blood-clotting disorder), and alcohol abuse. Importantly, the algorithm found these conditions hidden in language that may not pop up during a normal conversation with a doctor, noted H. Andrew Schwartz, Ph.D., an assistant professor of computer science at Stony Brook University and a study co-author.
“Our digital language captures powerful aspects of our lives that are likely quite different from what is captured through traditional medical data,” Schwartz said.
The team developed the algorithm based on the health records and Facebook status updates from 999 individuals (a total of over 20 million words). From there, the team started to develop a series of “word clouds” that appeared to be associated with each condition.
Some of the words in these clouds were fairly straightforward. Alcoholism, for instance, was associated with words like “drunk” or “bottle”; depression was discerned by words associated with physical and emotional discomfort, like “head,” “hurt,” “pain,” and “tears.” But there were also some far less intuitive language patterns.
Substance use disorder tended to have ties to hostile language in Facebook updates — in the paper, the team highlights words like “dumb” or “bullshit.” On the other hand, religious language had strong ties to diabetes. The patients who mentioned terms like “god,” “family,” and “pray” the most were 15 times more likely to have diabetes diagnoses than those who used less religious language.
A sample of word clouds from statuses that could reliably be used to predict diabetes, STDs, or drug abuse.
Used in tandem with demographic information, they were able to predict with “above chance” accuracy for all 21 conditions. But overall, the algorithm tended to really perform well when predicting rates of pregnancy, diabetes, and mental health conditions including psychoses, anxiety, and depression.
Lead study author Dr. Raina Merchant, the director of Penn’s Center for Digital Health, added that she hopes this information could be used to help patients manage conditions, or at least help doctors see things they might otherwise miss.
“For instance, if someone is trying to lose weight and needs help understanding their food choices and exercise regimens, having a healthcare provider review their social media record might give them more insight into their usual patterns in order to help improve them,” Merchant said.
But despite the promise of this analysis, it is a strange time to debut research that seeks to combine Facebook with information as intimate as a medical status. Facebook is under a federal privacy investigation and has been caught in the crosshairs of privacy concerns facing big tech since the Cambridge Analytica scandal. Inverse reached out to Facebook regarding the PLOS One paper’s findings and will update this article when we hear back.
"Our digital language captures powerful aspects of our lives that are likely quite different from what is captured through traditional medical data."
In contrast to some of Facebook’s practices, this research falls well within ethical boundaries — to start with, it was conducted on a sample of willing participants who knew that they were providing their social media information for medical purposes. And the authors are aware that these results don’t exist in a vacuum. They note that “the power of social media language to predict diagnoses raises parallel questions about privacy, informed consent, and data ownership.”
But now that we know how powerful this data may actually be, the authors are aware that continuing to inform patients will be important. Still, the potential to spot the origins of serious conditions highlights the big benefits of finding a morally acceptable way to work with people’s social media data on an even larger scale.
Abstract:
We studied whether medical conditions across 21 broad categories were predictable from social media content across approximately 20 million words written by 999 consenting patients. Facebook language significantly improved upon the prediction accuracy of demo- graphic variables for 18 of the 21 disease categories; it was particularly effective at predict- ing diabetes and mental health conditions including anxiety, depression and psychoses. Social media data are a quantifiable link into the otherwise elusive daily lives of patients, providing an avenue for study and assessment of behavioral and environmental disease risk factors. Analogous to the genome, social media data linked to medical diagnoses can be banked with patients’ consent, and an encoding of social media language can be used as markers of disease risk, serve as a screening tool, and elucidate disease epidemiology. In what we believe to be the first report linking electronic medical record data with social media data from consenting patients, we identified that patients’ Facebook status updates can pre- dict many health conditions, suggesting opportunities to use social media data to determine disease onset or exacerbation and to conduct social media-based health interventions.