A new AI system just confirmed 50 new planets
The method could save astronomers a lot of time when scouring the cosmos for life.
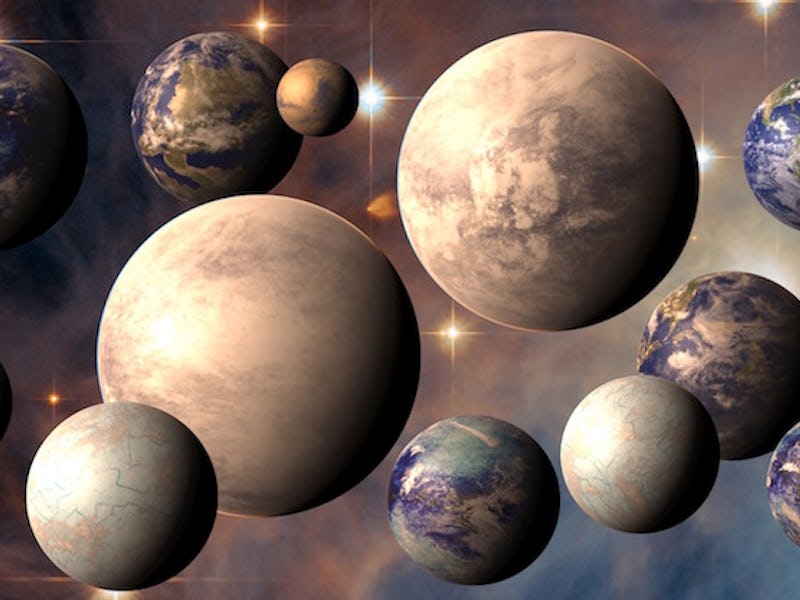
In 1995, astronomers Aleksander Wolszczan and Dale Frail were observing a distant pulsar star when they noticed that the regular rhythm of its pulsating light seemed to be skipping a beat.
The off- rhythm pulsating light led to the discovery of the first exoplanet, dubbed 51 Pegasi b. Since then, the business of finding odd and distant worlds orbiting stars other than our Sun has boomed, with more than 4,000 exoplanets confirmed so far.
The search is still on with ongoing space missions scouring the cosmos. To speed up the process, a team of astronomers has come up with a new machine-learning algorithm that rules out the false positives and confirms the real exoplanets.
With the help of their AI system, the team of astronomers from the University of Warwick have confirmed the existence of 50 new planets.
The new technique is detailed in a study published this week in the Monthly Notice of the Royal Astronomical Society.
A few examples of exoplanets that have been recently discovered by NASA's Kepler mission.
The two main missions behind ongoing exoplanet discoveries are NASA’s Kepler mission and the Transiting Exoplanet Survey Satellite (TESS) missions. Kepler zooms in on stars to see if there are planets orbiting within their habitable zone, while TESS surveys 200,000 of the brightest stars in the sky.
As a planet transits in front of its host star, it causes a slight dip in the star's light. Exoplanet hunters look for that dip as a clue that there may be a planet orbiting that star. But after the initial detection, astronomers then need to confirm it was in fact a planet causing the light variation.
There are more than 3,000 such blips waiting to be confirmed as planets, and not false positives.
David Armstrong, professor at the University of Warwick's department of physics, and lead author behind the new study, wanted to create an algorithm that would help astronomers sift through that data a lot faster.
"We wanted to develop this algorithm to work with ongoing missions," Armstrong tells Inverse.
"It's much faster and we can apply it to many more candidate planets."
The team of researchers trained the algorithm to recognize which planets are real and which are not by feeding it a large sample of confirmed planets and false positives. By spotting patterns in the data, the machine-learning system learned how to separate between the two categories.
Then the researchers used the algorithm on a dataset of thousands of unconfirmed planets, including ones gathered by NASA's Kepler mission.
The algorithm was able to confirm 50 new planets from the dataset. These planets range from Neptune-sized giants to ones closer to Earth in size, with orbits ranging from 200 days to one single day.
"What we've done differently than other machine learning techniques is trying to make it probabilistic, which ones are statistically likely to be planets rather than just ranking them," Armstrong says.
Previous machine-learning algorithms would rank exoplanets in terms of the chances of them being planets, but this new algorithm system determines the probability of each planet being a planet on its own.
"The 50 that came out were the ones that crossed the threshold of 99 percent probability of a planet," Armstrong says. "Those were the ones that crossed that line."
As new discoveries of exoplanets continue, the researchers will continue to feed the algorithm system more of these planetary candidates and it will progressively improve its skills. The faster it becomes to confirm real planets from fake ones, the faster we can follow up with these planets to search for habitability in the cosmos.
Abstract: Over 30% of the ∼4000 known exoplanets to date have been discovered using ‘validation’, where the statistical likelihood of a transit arising from a false positive (FP), non-planetary scenario is calculated. For the large majority of these validated planets calculations were performed using the vespa algorithm (Morton et al. 2016). Regardless of the strengths and weaknesses of vespa, it is highly desirable for the catalogue of known planets not to be dependent on a single method. We demonstrate the use of machine learning algorithms, specifically a gaussian process classifier (GPC) reinforced by other models, to perform probabilistic planet validation incorporating prior probabilities for possible FP scenarios. The GPC can attain a mean log-loss per sample of 0.54 when separating confirmed planets from FPs in the Kepler threshold crossing event (TCE) catalogue. Our models can validate thousands of unseen candidates in seconds once applicable vetting metrics are calculated, and can be adapted to work with the active TESS mission, where the large number of observed targets necessitates the use of automated algorithms. We discuss the limitations and caveats of this methodology, and after accounting for possible failure modes newly validate 50 Kepler candidates as planets, sanity checking the validations by confirming them with vespa using up to date stellar information. Concerning discrepancies with vespa arise for many other candidates, which typically resolve in favour of our models. Given such issues, we caution against using single-method planet validation with either method until the discrepancies are fully understood.