Our secret weapon against the next pandemic could be hiding in plain sight
Hint: It's in your pocket.
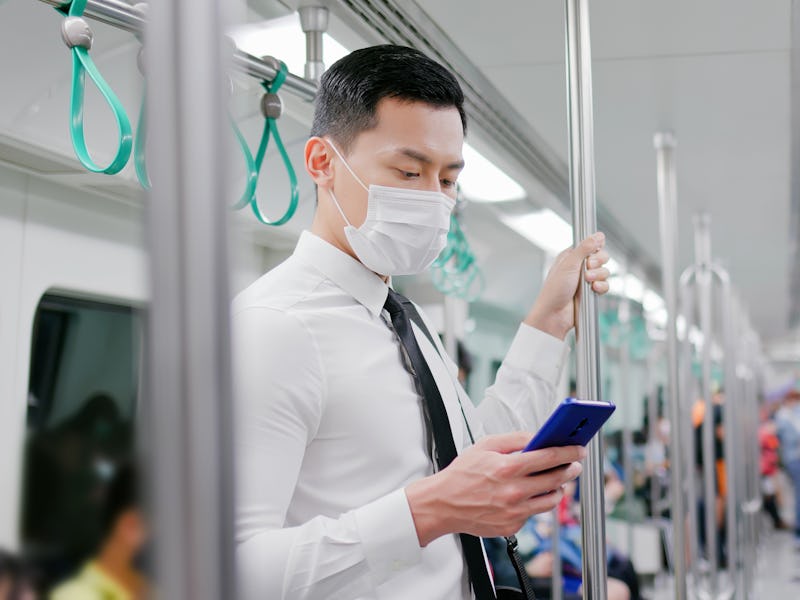
If 2020 taught us anything, it is that humans are excellent at spreading disease. But this same aptitude for being walking disease vectors may ultimately help fight the next Covid-19 pandemic.
Just as meteorologists can predict storm systems across the world by tracking cold fronts, so can epidemiologists predict the spread of infection through a densely populated city — by tracking our every move.
And what better means for tracking us than the homing beacon we keep in our pockets at all times?
Smartphones could be key to fighting the next pandemic, enabling researchers to predict spread through cities.
What's new — In a study published Tuesday in the journal Nature Communications, researchers demonstrate how location data from smartphones can accurately model infection through dense cities like New York, as well as sparsely populated countries like Australia.
Comparing their models to the "ground truth" data from the 2016-2017 influenza season, the researchers report that they were able to accurately capture infection spread with location data alone, essentially mapping pandemics as they grow.
The study is a collaboration between research universities and Google.
Why it matters — This kind of passive data, which our phones often generate automatically, could be a cost-effective and scalable approach to infection modeling. Ultimately, these data could help cities or even countries rapidly respond to impending outbreaks, and even stop them in their tracks.
And while these new models focus on the spread of flu, similar modeling methods could be adapted to emerging pathogens or pandemics, as well. Importantly, these models keep smartphone data private — a huge concern for privacy activists skeptical about handing over these kind of cellphone data to a public organization.
The big idea — Gathering data about peoples' daily commutes to model infection rates is nothing new, but it is typically done through either resource-intensive commuter surveys (to collect first-hand information on peoples' whereabouts,) accessing phone call records, or monitoring peoples' internet search history for queries related to illnesses.
The researchers behind this study point out that while these methods can be reliable, they're not necessarily doable in all cases, especially for cities or states with limited funds or infrastructure, or with more sparse populations.
NYC (left) and Australia (right) have very different population densities, but the researchers demonstrated that their disease model worked just as well in both environments.
Instead, the researchers take an automated approach. They use machine learning to collect and break down passive location history from smartphones into individual "trips." They then put their method to the test against real flu data from 2016-2017 from both New York City, and Australia.
What they did — The researchers first established a "ground truth" of flu infections from 2016-2017 using emergency room data and lab-tested positive flu results.
The scientists then compared four different disease models:
- Location history
- Commuter surveys
- Population size
- Population spread
The models were all trained on several weeks of ground-truth flu data, and then let loose to model the rest of the season's spread.
What they discovered — The location-history based model appeared to be better than those based on population statistics, and just as good at the commuter data, at estimating disease spread, according to the study. This was true in NYC, where the population is dense, and Australia, where the population (and thus, data) is more sparse.
Using location data, as opposed to phone records, also enabled them to track spread across state or city lines, providing a more holistic picture.
What's next — While these first two case studies provide promising evidence for how a location-based model could perform in comparison to more expensive, commuter-based models, the researchers say that there are still gaps in its reach.
Children and the elderly, for example, may be less likely to carry smartphones when they go out, making their impact on disease spread difficult to predict using this model. The model also struggles to separate residents from tourists, which can impact its ultimate accuracy.
In the future, the researchers write that solving these problems can help further strengthen their model and suggest that even combing models — for example, with commuter data — could create an even stronger-yet prediction model.
Abstract: Human mobility is a primary driver of infectious disease spread. However, existing data is limited in availability, coverage, granularity, and timeliness. Data-driven forecasts of disease dynamics are crucial for decision-making by health officials and private citizens alike. In this work, we focus on a machine-learned anonymized mobility map (hereon referred to as AMM) aggregated over hundreds of millions of smartphones and evaluate its utility in forecasting epidemics. We factor AMM into a metapopulation model to retrospectively forecast influenza in the USA and Australia. We show that the AMM model performs on-par with those based on commuter surveys, which are sparsely available and expensive. We also compare it with gravity and radiation based models of mobility, and find that the radiation model’s performance is quite similar to AMM and commuter flows. Additionally, we demonstrate our model’s ability to predict disease spread even across state boundaries. Our work contributes towards developing timely infectious disease forecasting at a global scale using human mobility datasets expanding their applications in the area of infectious disease epidemiology.
This article was originally published on