"Plug and play" brain prosthesis could change how people with paralysis use implants
“We see this as trying to build a partnership between two learning systems — brain and computer — that ultimately lets the artificial interface become an extension of the user, like their own hand or arm."
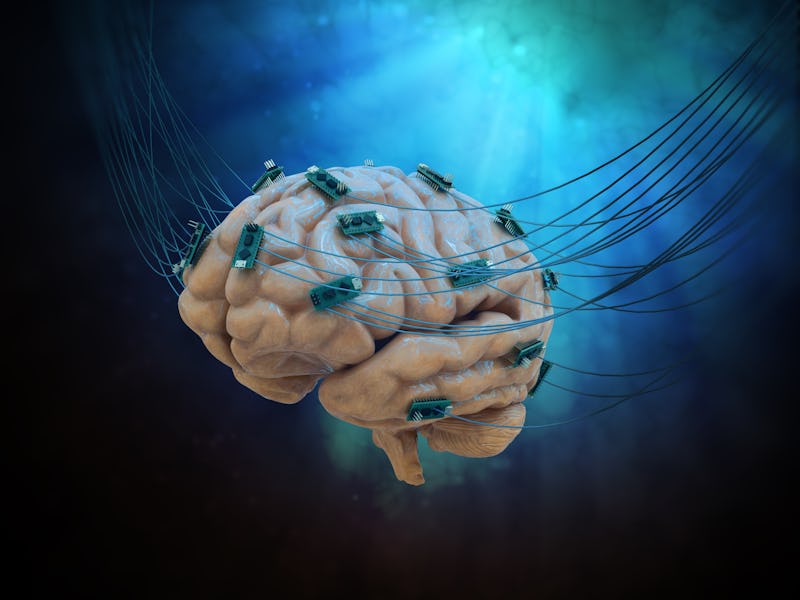
Imagine trying to learn a new skill but waking up the next day always forgetting most of the progress you made.
For paralyzed individuals relying on brain-computer interfaces (BCIs) to help regain control of certain limbs, this can be a frustrating reality.
BCIs, not unlike the Neuralink implant designed by Elon Musk, have made great advancements in recent years, but an intrinsic drawback of these systems is that they have to be reset daily, which disrupts the brain's natural learning process.
Neurologists from the University of California, San Francisco set out to see if they can break this cycle.
They have conducted a single-patient study to see how a BCI would fair without recalibrating daily. They found that forgoing recalibration not only worked, but it led to more natural neural map creation, similar to muscle memory, and also the ability to add new skills as well.
"It’s like asking someone to learn to ride a bike over and over again from scratch."
In the study, published Monday in the journal Nature Biotechnology, the researchers describe the difference between two popular types of BCIs: 128-channel chronic electrocorticography (ECoG) implants and a more standard implant pin-cushion style intracortical implants that penetrate the brain tissue.
While the pin-cushion style is traditionally more sensitive, and researchers argue that its effectiveness is limited by its need for daily recalibration. ECoG implants, on the other hand, may be a solution for longer-term use, they say.
"The BCI field has made great progress in recent years, but... they haven’t been able to tap into the brain’s natural learning processes. It’s like asking someone to learn to ride a bike over and over again from scratch," senior author on the paper and associate professor in of neurology at UCSF, Karunesh Ganguly, said in a statement.
“Adapting an artificial learning system to work smoothly with the brain’s sophisticated long-term learning schemas is something that’s never been shown before in a paralyzed person."
The researchers explain that this solution could offer "plug and play" performance for these BCIs, meaning there would be less hassle and upkeep to begin using them.
To test out these hypotheses, the team worked with one individual with tetraplegia over the course of six months.
"We see this as trying to build a partnership between two learning systems—brain and computer."
HOW THEY DID IT — The researchers began by giving the participant two months to recover from implantation surgery before they began introducing him to the movement task at hand — controlling the motion of a computer cursor by sending electric signals to the implant.
A machine-learning algorithm then translates these signals into movement of the cursor. For the participant, this simply meant imagining specific wrist and hand movements.
To start, the researchers continued the standard practice of recalibrating the implant at the end of each day. As expected, they found that this was detrimental to the level of performance the participant could achieve over time. They report that it could take hours each day to gain mastery over the device, and that sometimes the participant had to give up altogether for lack of progress.
So, in the second half of the experiment, they implemented a new learning technique that allowed the machine learning algorithm to better learn and develop a neural map. How? By simply not resetting the device.
They found that this approach resulted in continuous improvement over many days and recovery of what little loss progress there might be. They also found that the progress trajectory continued even if left untrained for several days.
“We found that we could further improve learning by making sure that the algorithm wasn’t updating faster than the brain could follow — a rate of about once every 10 seconds,” said Ganguly.
“We see this as trying to build a partnership between two learning systems — brain and computer — that ultimately lets the artificial interface become an extension of the user, like their own hand or arm."
A photo of the type of brain implant used in this study.
The researchers also observed that the participant's brain refined its neural map of the process over time by amplifying effective pathways and "pruning" ineffective ones.
The team also observed that this learning model was resilient and, even after the learning cycle was reset a few weeks later, the participant's brain and device again quickly converged on previous solutions and models. This is evidence of an "enduring memory" says Ganguly.
The participant was able to go 44 days without retraining the implant, and was even able to add additional skills, like "clicking" a virtual button without losing progress.
What's next — While ECoGs, like the researchers used here, and less sensitive and in turn less frequently used than other methods, the researchers say that the results of this study demonstrate how they might actually be a solution for a long-term BCI. This current research was done on a single person, but the team writes they're excited to see how such an approach would play out in a larger population as well. Ultimately, said Ganguly, reaching as many people as possible with this technology is the goal.
“We’ve always been mindful of the need to design technology that doesn’t end up in a drawer, so to speak, but which will actually improve the day-to-day lives of paralyzed patients,” Ganguly said. “These data show that ECoG-based BCIs could be the foundation for such a technology."
Abstract: Brain–computer interfaces (BCIs) enable control of assistive devices in individuals with severe motor impairments. A limitation of BCIs that has hindered real-world adoption is poor long-term reliability and lengthy daily recalibration times. To develop methods that allow stable performance without recalibration, we used a 128-channel chronic electrocorticography (ECoG) implant in a paralyzed individual, which allowed stable monitoring of signals. We show that long-term closed-loop decoder adaptation, in which decoder weights are carried across sessions over multiple days, results in consolidation of a neural map and ‘plug-and-play’ control. In contrast, daily reinitialization led to degradation of performance with variable relearning. Consolidation also allowed the addition of control features over days, that is, long-term stacking of dimensions. Our results offer an approach for reliable, stable BCI control by leveraging the stability of ECoG interfaces and neural plasticity.