Tracking disinformation using "digital fingerprints"
Can you tell a bot from a human?
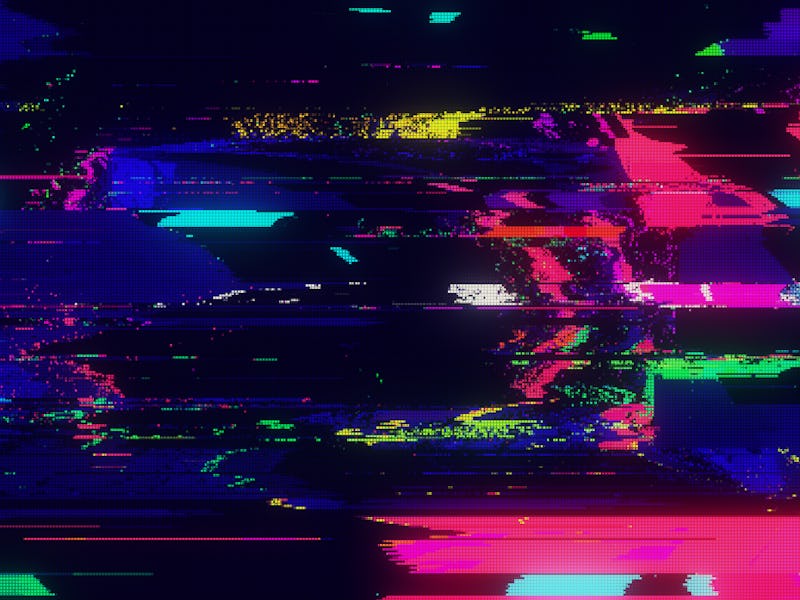
Getting in a Twitter flamewar is the quickest way to lose your humanity, and send your anxiety skyrocketing. Twitter trolls, after all, often use a unique mixture of empathy and psychopathy to send your tensions through the roof. But what if the account you're angry about isn't even human at all?
Bots and coordinated foreign influence campaigns have infiltrated our social media feeds in recent years and driven division, but researchers now have a new way to spot and squash these trolls using machine learning.
What's the news -- In a new study published Wednesday in the journal Science Advances, an interdisciplinary team of computer and social scientists from Princeton has developed a new approach to reliably identifying coordinated influence campaigns on Twitter and Reddit. More than just angry or nonsensical bots, these campaigns can have dangerous repercussions for geopolitics, including Russia's recent tampering in the 2016 U.S. election.
By being able to reliably identify the traits of a social media influence campaign, down to the posts' word count and timing, it would be possible to identify and disrupt these campaigns before they begin to leach into the general public.
But first, the team had to ask one important question: what distinguishes troll activity from everyday users?
Foreign disinformation campaigns are designed to spread division online and mimic human posting behavior.
How does it work -- In their study, the researchers focused on three historical influence campaigns from Russia, China, and Venezuela, and looked specifically at the activity on these campaigns on Twitter and Reddit. They trained a machine-learning algorithm on 7.2 million posts from these countries between late 2015 through 2019, as well as posts from politically engaged U.S. users and casual U.S. users.
While it might be easier for the algorithm to simply trace posts' I.P. addresses to track their origin, in order to truly determine the difference between influence posts and "regular" posts, they focused instead on only using content-based features to identify them. For example, what kind of URLs did the posts link to, how many characters did they use, what time did they post, and who did the posts commonly interact with.
More than just discrete features, the algorithm was able to see connections between these features and use a kind of "metacontent" to replicate human intuition. For example, it looked at whether a URL was among the top 25 URLs shared by trolls.
But, instead of looking at all of this information at once and making decisions, the study's first author and a computational social scientist at Princeton, Meysam Alizadeh, tells Inverse that they instead looked at the data one month at a time in order to simulate a more realistic experience.
"We decided to simulate the real world," says Alizadeh. "The question is, had we had this system back in 2016... and a given set of data of Russian, Chinese or Venezuelan operations on social media, could we find the rest of the activity in each month."
What were the results -- Using their content-based machine learning algorithm, the team discovered a few key differences between these three campaigns. First, they saw that the Venezuelan campaign was much easier to tell apart from casual Twitter use than those of the Russian or Chinese campaigns. The authors mostly attribute this to the fact that the Venezuelan trolls only retweeted certain topics or people. Alizadeh tells Inverse that the algorithm identified these posts with nearly perfect accuracy.
"We want a public dashboard to show whether or not there was an information operation behind [a story,] regardless of whether [it] is true or false."
The Russian and Chinese campaigns, on the other hand, were much better at mimicking human-like social media behavior. But, between these two, Chinese campaigns were still easier to detect (with about 90 percent accuracy) than Russian ones (with 85 percent accuracy.) All this, Alizadeh tells Inverse, with a metaphorical hand tied behind their back thanks to the content-only identifying method.
While the authors write that there is not necessarily one set of characteristics that identify these posts, they are still highly detectable nonetheless -- the algorithm was even able to identify never-before-used accounts that were part of an ongoing campaign. But of course, the problem becomes more complex as new campaigns emerge and these campaigns change their approach.
What's next -- The authors write that an algorithm like theirs could be employed by social media sites to quickly identify these campaigns. While deleting or blocking the accounts would be one way to disrupt the campaign, the authors worry that this might counterproductively work to alert the trolls that they've been caught and in turn force them to create new, harder to identify campaigns. An alternative could be instead to silo the posters and trick them into thinking their posts are still receiving interaction.
Going forward, Alizadeh says he's also interested in expanding the scope of this approach to include social media influence campaigns happening in non-English speaking countries and to images, which could play a big role in future campaigns in the form of memes.
Alizadeh says he also hopes to develop better transparency between social media platforms and their users.
"I'd like to have a public dashboard so that journalists and the public can check that dashboard on a daily basis to see what are the top stories on U.S. social media and whether or not there was an information operation behind that," says Alizadeh. "[This] doesn't mean that we want to show the content of the Russian trolls, we just want that public dashboard to show whether or not there was an information operation behind this, regardless of whether that story is true or false."
Abstract: We study how easy it is to distinguish influence operations from organic social media activity by assessing the performance of a platform-agnostic machine learning approach. Our method uses public activity to detect content that is part of coordinated influence operations based on human-interpretable features derived solely from content. We test this method on publicly available Twitter data on Chinese, Russian, and Venezuelan troll activity targeting the United States, as well as the Reddit dataset of Russian influence efforts. To assess how well content-based features distinguish these influence operations from random samples of general and political American users, we train and test classifiers on a monthly basis for each campaign across five prediction tasks. Content-based features perform well across period, country, platform, and prediction task. Industrialized production of influence campaign content leaves a distinctive signal in user-generated content that allows tracking of campaigns from month to month and across different accounts.