13,000
Can your data today predict your future?
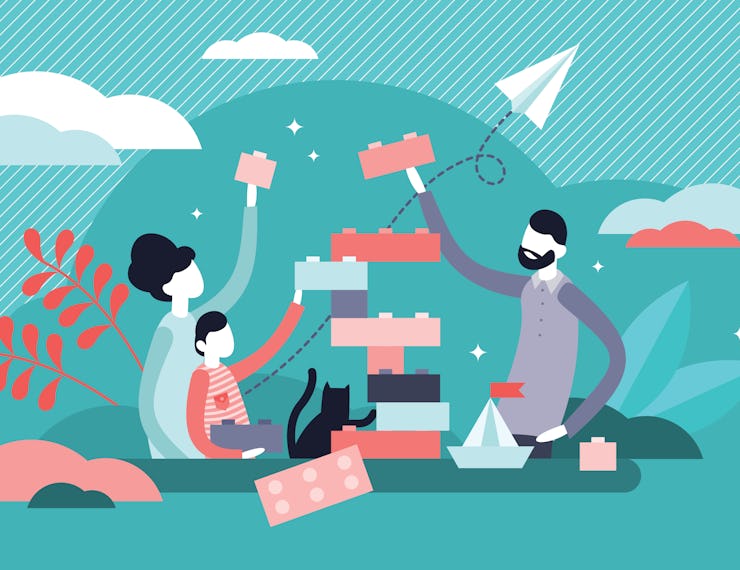
Can AI accurately predict the rest of your life?
A massively collaborative research effort has looked at 15-years worth of data and determined that AI is no good at predicting our futures.
by Sarah WellsThe data we generate every day leave behind a digital footprint that defines our daily lives, but can that same data be used to predict our futures? In a surprising win for free will, researchers say no.
Examining over 13,000 data points collected in a 15-year-long study on children and families, 160 research teams running machine learning models found that they were unable to accurately predict the lives of children when looking at six-variables, including GPA and family eviction. Researchers say that these results throw into question long-held theories on childhood development.
Co-lead author and professor of sociology at Princeton, Matt Salganik, said in a statement that these results show that just because machine learning tools may be powerful, doesn't mean they're invincible.
"These results show us that machine learning isn't magic; there are clearly other factors at play when it comes to predicting the life course," said Salganik. "The study also shows us that we have so much to learn, and mass collaborations like this are hugely important to the research community."
The paper, published on March 30 in the journal PNAS, is the result of a study which lasted over 15 years, conducted by Princeton and Columbia on the wellbeing of children and families. Beginning in the late 90s, the study has followed over 4,000 families from the time they gave birth to their children until those children turned 15. Throughout this period, the study collected data in six different waves (including birth, ages 1, 3, 5, 9 and 15) and asked children and their caregivers questions about the family's financial and marital status and the accomplishments of the children.
The authors of the machine learning study write that this wealth of data provided a perfect opportunity to test the robustness of their prediction models. The teams trained their models on released data from the study's first five waves and half of the sixth wave's data.
In theory, this training should have then allowed their models to make fairly accurate predictions about what the second half of the sixth wave data would look like, particularly concerning "the child's grade point average (GPA); child grit; household eviction; household material hardship; primary caregiver layoff; and primary caregiver participation in job training."
But the models were actually really bad at making these predictions.
When comparing results from these hundreds of different prediction models to the actual second half of the data, the researchers found that the models were accurate 20 percent of the time when predicting material hardship and GPA and accurate only 5 percent of the time in the other four categories.
Sara McLanahan, professor of sociology and public affairs at Princeton and co-author of the study, said in a statement that these results suggest that there may be serious gaps in our sociological understandings of childhood development.
"The results were eye-opening," said McLanahan. "Either luck plays a major role in people's lives, or our theories as social scientists are missing some important variables. It's too early at this point to know for sure."
That said, the authors write that policymakers who rely on predictive models like these in their work, such as criminal justice enforcers or child-protective services, should be concerned about the results of this study and think more carefully about what the negative impacts of these false predictions might be on those on the receiving end.
Abstract: How predictable are life trajectories? We investigated this question with a scientific mass collaboration using the common task method; 160 teams built predictive models for six life outcomes using data from the Fragile Families and Child Wellbeing Study, a high-quality birth cohort study. Despite using a rich dataset and applying machine-learning methods optimized for prediction, the best predictions were not very accurate and were only slightly better than those from a simple benchmark model. Within each outcome, prediction error was strongly associated with the family being predicted and weakly associated with the technique used to generate the prediction. Overall, these results suggest practical limits to the predictability of life outcomes in some settings and illustrate the value of mass collaborations in the social sciences.