Scientists Say 1 Habit Separates Amateurs From eSports Pros
You might want to sit up for this one
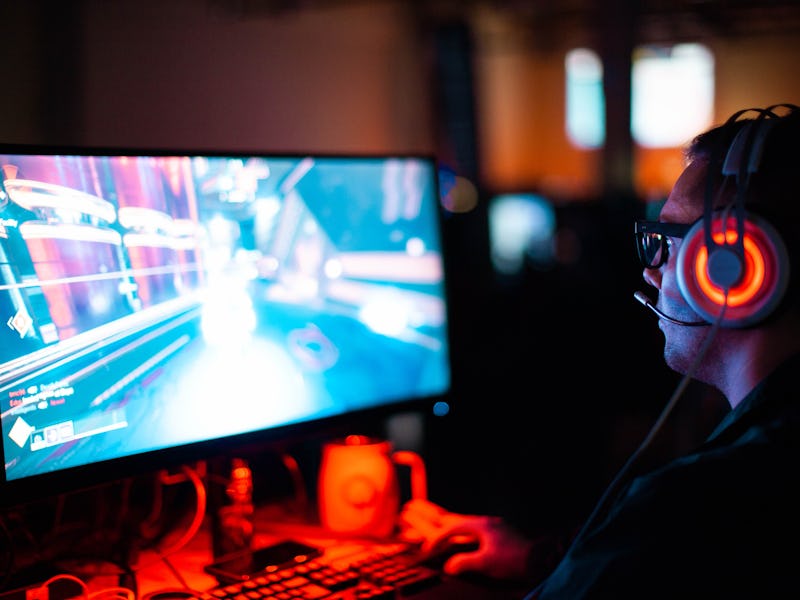
Who knew Counter-Strike could be so beneficial to science? With eSports continually rising in worldwide relevance, a team of researchers in Russia has taken a closer look at what exactly separates a professional player from amateurs.
In a study presented at the IEEE’s 5th World Forum on Internet of Things this spring, the team of scientists at Skoltech, the Moscow Institute of Physics and Technology, and the State University of Aerospace Instrumentation in St. Petersburg trained a machine learning algorithm to correctly distinguish the movement patterns of a professional player from an amateur 77 percent of the time. Their results showed that a player’s posture tells you almost everything you need to know.
The team reached this conclusion by looking at how 19 players — nine professionals and 10 amateurs — moved in their chairs while playing 30- to 60-minute sessions of Counter Strike: Global Offensive and analyzed their movements via an accelerometer and a gyroscope that were physically embedded in the players’ chairs. For the sake of the study, the researchers determined levels of professionalism based on the number of hours of a game play a player had completed, with a higher number of hours completed equaling more professional players and a lower number equaling less professional ones.
An accelerometer and a gyroscope were embedded in each player's chair.
Anton Smerdov, the study’s first author and a graduate student at Skoltech and MIPT, said the team suspected players’ movements would reveal surprising amounts of information about their skills.
“We hypothesized that there could be a link between a player’s body movements and skill level,” he explained in a press release. “Also, it was interesting to look at the players’ responses to various game events, such as kills, deaths, or shootings. We suspected that professional players and beginners would react differently to the same event.”
To determine the validity of this hypothesis the team cut the nearly hour-long game play into three-minute chunks and broke each player’s movements down into 31 discrete patterns — how they moved around the three axes of the chair, to the front, sides or back. From there, they narrowed those down to eight key movements each that could be fed to a machine learning algorithm as input.
Posture separates the amateurs from the professionals.
Using all the three-minute chunks, the team trained the algorithm to correctly identify the differences between a professional and an amateur player. They found that professional players more characteristically made fewer active movements during game play in comparison to their amateur counterparts. The movements they did make included slow, parallel movement along the table and active movement in the z-direction aka sitting up. In their study the researchers suggest that these less active movements may reflect a higher level of concentration in professional players.
Comparatively, the study showed that amateur players were more likely to lean back in their chairs and make more active rotation in their chair overall.
These findings also contribute to previous research from the team which looked at the heart rate of players, their gaze direction, and hand movements, among other parameters. The researchers write that this kind of deeper look into the habits of professional eSports athletes will help future players train and succeed in this arena.
Abstract:
eSports is the rapidly developing multidisciplinary domain. However, research and experimentation in eSports are in the infancy. In this work, we propose a smart chair platform - an unobtrusive approach to the collection of data on the eSports athletes and data further processing with machine learning methods. The use case scenario involves three groups of players: cyber athletes (Monolith team), semi-professional players and newbies all playing CS:GO discipline. In particular, we collect data from the accelerometer and gyroscope integrated in the chair and apply machine learning algorithms for the data analysis. Our results demonstrate that the professional athletes can be identified by their behaviour on the chair while playing the game.