Nightmare Images Created by Monkey Brains Solve a Mystery About How We See
"They looked like objects in the world that were not in the world."
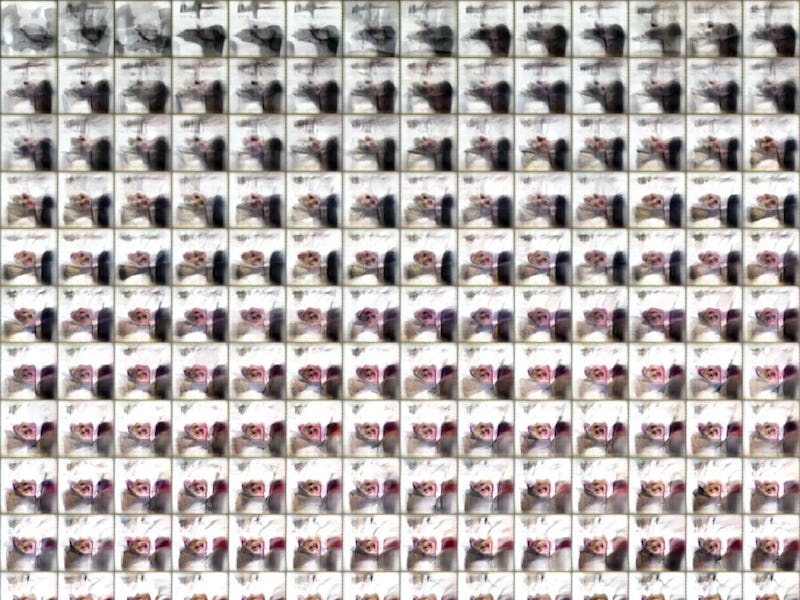
Every time you look at a face, a group of neurons behind your ears goes wild with excitation. For a long time, scientists have pondered what it is, exactly, that tickles the very particular fancies of these neurons. Is it a certain eyes-nose-mouth combination that triggers its frenzy? A particular arrangement of colors? What is a face, to a neuron? In a groundbreaking Cell study, scientists found out through an unusual approach: They asked the cells themselves.
"They looked like objects in the world that were not in the world."
“We’ve been stuck with this problem for decades,” first author Carlos Ponce, Ph.D., a neuroscientist at Washington University School of Medicine in St. Louis, tells Inverse. Scientists trying to understand this aspect of our visual systems are trying to understand how it is we evolved to not only see but also recognize complex images like faces, and also objects, places, and animals. Previously, researchers investigated this by showing subjects countless images to find out what was best at turning their neurons on — an impossible task, since there are an infinite number of images to show.
To do the impossible, Ponce and his team took advantage of a powerful new tool. They turned to a type of A.I. used to generate imaginary but uncannily realistic images like DeepFakes and other creepy art. These generative adversarial networks, or GANs, evolve images based on input from a “discriminator” that determines what’s good and what’s not. In Ponce’s experiments, the discriminator was the monkey neuron, hooked up to the GAN, which burst with activity if it approved of the image it saw. As the images evolved, one thing became clear: These cells are into some weird shit.
“They looked like objects in the world that were not in the world,” says Ponce.
This "evolved" image is what maximally stimulated one monkey's neurons.
When Reality Isn’t Enough
This neural network, called XDREAM, took real-time information about the excitation of a neuron and used it to shape increasingly stimulating images.
There are neurons specific to faces, but there are also some that prefer animals, and others objects. To identify each neuron’s specificity, the authors first showed the animals arrays of different photos, like those shown in the right column below, and watched for their activity. When that was established, it was the cell’s turn to create its dream image.
The images on the left are what the GAN produced in response to the activity of face-recognizing neurons. They resemble dream-like faces. On the right are the "natural images" that the scientists used to identify the face neurons in the first place.
"We found that these cells were responding better to … the dream versions of these natural-world pictures."
The monkeys started off by looking at a black and white texture — to keep it “non-biased,” says Ponce — that evolved, ever so slightly, as the neural network responded to the changes that made its neurons fire the most.
Likening the image-generating process to human reproduction, Ponce said that the most stimulating pictures “had sex” with one another to create increasingly stimulating offspring. The neurons responded well to some mutations and not to others, and as the GAN learned from its mistakes and produced increasingly more stimulating pictures, the scientists began to recognize familiar objects through the fuzz over one to three hours, depending on how long the monkey could sit still.
In some instances, the evolved images revealed “features of animal faces, bodies, and even animal-care staff known to the monkeys,” the team writes. But when they looked closer, the team realized the images resembled real-life objects but didn’t seem quite right.
A face-like image emerges over the course of several image evolutions.
This was surprising. “We’re used to thinking that these cells are used to responding to very realistic depictions of the world,” says Ponce. “But in fact, we found that these cells were responding better to — I guess if I can use poetic license — the dream versions of these natural-world pictures.”
The specificity of each neuron’s tastes was spooky. One cell that was known to respond to faces, for example, did seem to choose a face-like image, with black spots resembling eyes and a complex border suggesting the outline of a head. “But it wasn’t a photorealistic face,” says Ponce. “It was something more abstract — something like the neuron’s interpretation of what a face looked like.”
How We Learn to See
When an infant sees a human face, it’s immediately drawn to it. This we know from research, as well as from experience. Some things — smiling faces, shiny things, the color red — are just irresistible to babies. With Ponce’s discovery, we’re getting a better understanding of what our brains are primed to recognize and what we must learn to see.
"There’s a certain kind of creepy overstimulation where my brain is trying to catch up with the images in front of me."
Ponce’s work has been an effort to decipher the “vocabulary” of the visual cells — the fundamental elements they recognize as stimuli. Previous work had suggested that a cell might respond to something as concrete as a face, but the images show this is clearly not the case. “We thought that it was things like photorealistic textures, but in fact it’s something much more abstract,” he says.
“It felt like for the first time we were finally communicating with an entity that hadn’t had a voice and now had some kind of voice to communicate back to us what it was that it’s trying to see.”
Experience, for one, must play a role in shaping the neuron’s preference — how else would they have evolved images that clearly resembled things from the monkeys’ lives, like the care staff with their familiar face masks, which visited them every day? And yet, since the neurons didn’t evolve images of faces as we know them, perhaps their so-called vocabulary is looser than we thought, or perhaps it is not even fixed.
“Maybe what we have is that evolution did not build in a template or a set of templates for objects but rather it built it in a very adaptive, very flexible learning mechanism that will then learn everything around it,” Ponce says.
One thing was clear: These images, self-selected by the neurons to be optimally stimulating, eventually became super-stimulating. Ponce has experienced this himself, while watching GAN-created videos like the one above.
“There’s a certain kind of creepy overstimulation where my brain is trying to catch up with the images in front of me,” he says. “I can imagine the monkeys might be feeling a little bit like that.”
Abstract:
What specific features should visual neurons encode, given the infinity of real-world images and the limited number of neurons available to represent them? We investigated neuronal selectivity in monkey infero- temporal cortex via the vast hypothesis space of a generative deep neural network, avoiding assump- tions about features or semantic categories. A genetic algorithm searched this space for stimuli that maxi- mized neuronal firing. This led to the evolution of rich synthetic images of objects with complex combi- nations of shapes, colors, and textures, sometimes resembling animals or familiar people, other times revealing novel patterns that did not map to any clear semantic category. These results expand our conception of the dictionary of features encoded in the cortex, and the approach can potentially reveal the internal representations of any system whose input can be captured by a generative model.