Google and Elon Musk's Open A.I. Tag-Team Against an A.I. Rebellion
The robot apocalypse won't be arriving just yet.
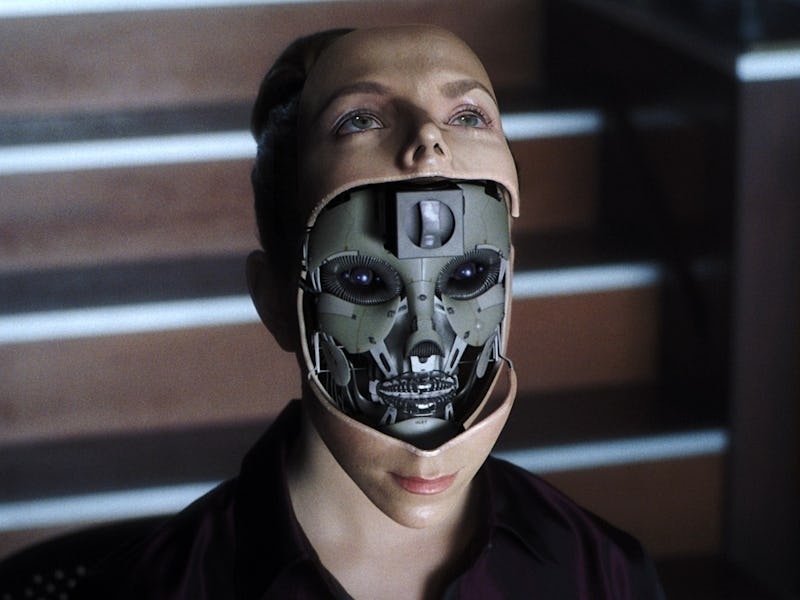
Artificial intelligence is poised to be the biggest industry of the world later this century, but today, it’s a relatively small world of individual research projects: There are competing businesses as well as university-based endeavors. But two of the field’s biggest names have partnered to solve a problem on the minds of anybody who’s thinking about the future of an A.I. robot rebellion.
Google DeepMind and the Elon Musk-associated OpenAI have released a new collaborative study this month which seeks to address how autonomous systems don’t rebel.
The new paper, currently unpublished but available for viewing on the arXiv repository, demonstrates an approach to teach A.I. systems how to learn new tasks using human-mediated directions and teachings as opposed to allowing a system to teach itself. The new model is designed to clamp down on unpredictable and surprising moves on the A.I.’s part.
The killer robots of last century's science fiction.
The new study revises a machine learning approach called reinforcement learning, in which software tests out a myriad of potential solutions to a certain task and begins to fine tune the best ones based on a reward structure. This is exactly how DeepMind’s AlphaGo program managed to learn how to thrash the hell out of so many human world champion Go players.
Unfortunately, reinforcement learning based on a reward function is not exactly an easy approach. An A.I. system might flounder when it comes to accomplishing an insanely difficult task. Or it may choose to find a shortcut that essentially defeats the purpose of the task (for example, as Wired observes, OpenAI once used reinforcement learning to teach an agent how to play a boat racing game, and the system figured out a way to score points by driving in circles rather than actually racing through the course.
So, DeepMind and OpenAI decided to see whether A.I. software would work better if it were utilizing human feedback. A simulated robot called a Hopper learned how to do a backflip after processing 900 different verdicts from human trainers as it attempted different movements. In 45 minutes, the Hopper learned how to land a very elegant backflip as opposed to the awkwardly shaped backflip accomplished over the span of two hours using conventional reinforcement learning.
Beyond this, the study suggests it might be more prudent for A.I. researchers to take a more active, intervening approach in teaching and advancing A.I. systems. There will always be a huge concern that A.I. could quickly deviate from their expected functions — especially in ways that could threaten the safety of humans. The solution, it seems, might simply be for humans to engage more directly with A.I. and provide, how you might say, a human element.