How A.I. Exoskeletons Could Make People Super-Human
Smart exoskeletons can help someone jump really high or relearn to walk.
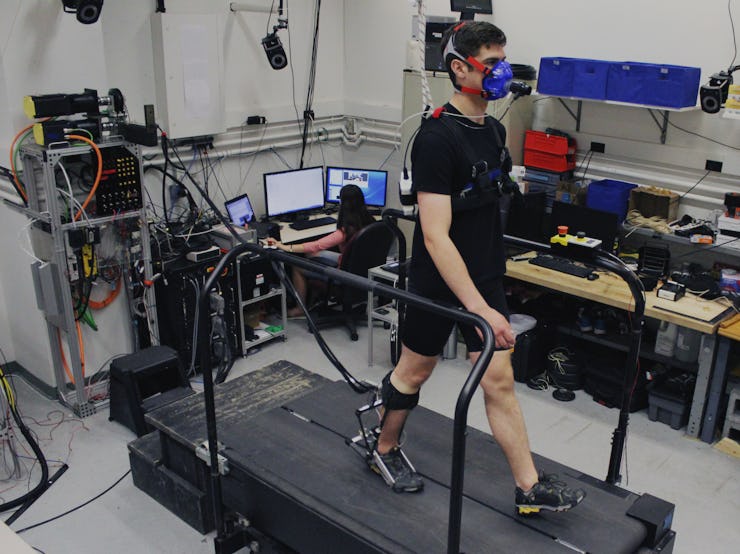
Robotic exoskeletons have been heralded as the future of industry, physical rehabilitation, and geriatric assistance, but progress has been slow. That may be changing, beacause researchers from Carnegie Mellon University in Pittsburgh are developing a machine learning algorithm that enables an exoskeleton to learn how to customize its movement for each individual. It’s an exoskeleton with a brain.
“I think the applications can be really wide,” Kirby Witte, a mechanical engineer and a Ph.D. candidate at Carnegie Mellon, tells Inverse. “They can be really awesome like having someone jump real high or kick down a door, or really significant like helping someone walk after a stroke.”
Part of the problem with exoskeletons up to now has been that they were hand-tuned, with researchers taking their best guess at how they should assist people, but research published this week in the journal Science works to solve that problem.
Witte explains that exoskeletons show a lot of promise, but the models and simulations that people use to program them are insufficient compared to real human data.
“In simulation work, we expect exoskeletons to significantly reduce energy use, but they don’t. And some of the reason they don’t is because the people are poorly modeled,” Witte tells Inverse.
To test their system, the researchers put people on a treadmill. One ankle was unassisted, and the other was connected to an exoskeleton brace that would apply a torque to help propel the person’s foot forward. Initially, researchers programmed the ankle brace according to their best guess as to when it might best help a person. Subsequent tests showed that the optimal timing varied by person.
Using an ankle brace to help walk was just a test run. The researchers have loftier goals.
“The way we intended to assist them was that we sensed when their foot touched the ground, waited some time, and applied a peak of torque.” Witte tells Inverse. “[Optimal] timing seemed to vary but it happened a little later than we expected.” It turns out that the most effective exoskeletons should apply a force on the person they’re assisting only after they begin to push on their own.
Witte says that this technology is still a couple years away from any clinical application, largely because hospitals and clinicians aren’t equipped with the right hardware and testing apparatus to use their algorithm in a meaningful way. However, Witte expects that her research would prove useful to people in physical therapy.
This research on energy reduction while walking is more of a test run for the algorithm, Witte says. Beyond that, a machine learning algorithm like hers could be used for any sort of human-robot interface.
Abstract:
Exoskeletons and active prostheses promise to enhance human mobility, but few have succeeded. Optimizing device characteristics on the basis of measured human performance could lead to improved designs. We have developed a method for identifying the exoskeleton assistance that minimizes human energy cost during walking. Optimized torque patterns from an exoskeleton worn on one ankle reduced metabolic energy consumption by 24.2 ± 7.4% compared to no torque. The approach was effective with exoskeletons worn on one or both ankles, during a variety of walking conditions, during running, and when optimizing muscle activity. Finding a good generic assistance pattern, customizing it to individual needs, and helping users learn to take advantage of the device all contributed to improved economy. Optimization methods with these features can substantially improve performance.